UNDERGRADUATE CAPSTONE
Bioengineering undergrads can participate through annual capstone projects that highlight mHealth design, development, and implementation. Recent projects include:

KNEE RANGE OF MOTION SENSOR
After knee replacement surgery, patients undergo physical therapy to ensure restoration of the full range of motion (ROM) during the healing process. This process prevents excess scar tissue from forming. Previously, patients undergo post-operative recovery and physical therapy at the clinic and were billed in a pay-for-service model. However, new Bundled Payments for Care Improvement (BPCI) has changed Medicare funding so that patients are now charged a “lump sum” up-front for all costs. This model has resulted in a push to discharge patients quickly for recovery at home – and thus, there is great interest in monitoring systems that can track the patients’ knee ROM to ensure proper recovery.
The UCLA Center for SMART Health sponsored a BE student capstone to develop a light weight, easy-to-use, and cost-effective ROM sensor to collect relevant data to assess knee motion during recovery. A ROM sensor for the knee and integrated software platform tracked patient compliance to post-op physical therapy exercises, providing real-time feedback to patients and healthcare providers. The stored data of the patient’s movement can then be used to visualize progress and develop more tailored physical therapy routines.

VOICE-ASSISTED PROS
As efforts continue to better integrate patients and their perspectives into decision-making at all levels of the healthcare system, patient-centered outcomes research (PCOR) is an increasingly important field of investigation. One valuable tool for incorporating the patient’s perspective is the collection of patient-reported outcomes (PROs), which are provided directly by patients without interpretation by providers. The Patient-Reported Outcomes Measurement Information System (PROMIS) was developed with support from the National Institutes of Health to enhance PRO collection. PROMIS had three broad objectives: 1) creating a large group of item banks measuring PROs; 2) developing a computerized adaptive testing (CAT) system that allows for efficient, psychometrically robust assessment of PROs; and 3) producing a publicly available online data collection system that allows clinical researchers to access the item banks and CATs.
With the advent of voice assistant technology, there is an opportunity to better integrate the collection of patient centered outcomes collection in more efficient and meaningful ways. The UCLA Center for SMART Health sponsored a BE capstone project focused on integrating PROMIS with voice assistant technology to assess: 1) usability and validity of voice assistant technology to collect structured data; 2) compare challenges and limitations of voice-assistant technology; 3) pilot test a model where patients prompt patient-centered outcomes data collection; and 4) use a context-based system fed by sensor information to prompt patient-centered outcomes data collection.
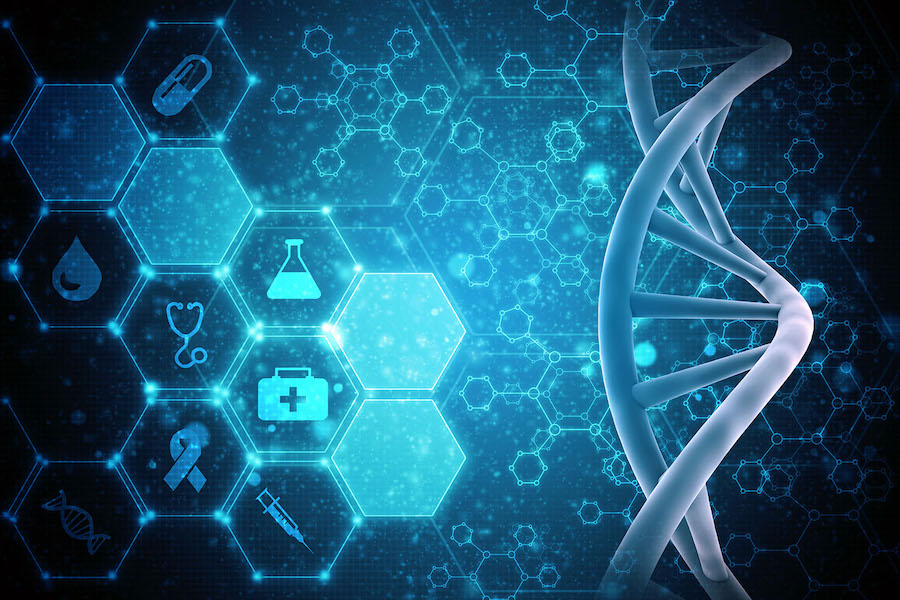
PREDICTING CANCER HISTORY
In this project, we sponsored a Bioengineering student capstone project that studied automated approaches to predicting if someone ever had cancer and examined the factors associated with such a prediction. This approach can be useful for determining a patient’s health history in situations where patients are reluctant to disclose such information. Data from the National Health and Nutrition Examination Survey (NHANES) collected between 1999-2016 were used. Features included a range of health-related and demographic variables, which were explored using heatmaps. To predict a person’s history of cancer, the students ran different supervised machine learning (ML) classification methods, and plotted calibration curves. Wilcoxon, Kruskal-Wallis, and chi-square tests were performed to find features that differed significantly between individuals with and without a history of cancer. ExtraTrees Classifier, AdaBoost Classifier, and Gradient Boosting Classifier performed the best at predicting history of cancer, with accuracy, precision, recall, and F1-scores of around 70%. Features which differed significantly between individuals with and without a history of cancer were age, systolic blood pressure, waist size, general self-reported health, household size, and pulse.